Once a behaving organism has acquired, processed, and transmitted sensory information it must still alter patterns of motor activation in a manner that enables control. A central challenge in understanding motor control, particularly during complex rhythmic behaviors like flight, is decoding the specific features of body dynamics that are control by patterns of neural feedback to different muscles. I am currently developing computational techniques for extracting relevant motor features and testing if groups of muscles have independent control authority or behave as a single “control knob” for the nervous system. To accomplish this, I have adapted reverse correlation approaches from sensory neuroscience and extended them to consider any number of continuous variables that describe the neuromuscular activation patterns (e.g. spike timing). I use a projection onto latent structures (PLS or partial least squares) approach to iteratively find linear combinations (features) of the torque that maximally predict what the motor commands were during each wingstroke. I then determine how well different subsets of muscle timing variables (e.g. the phase of each muscles spikes or only the phase difference) explained these these features. If only the timing difference between the muscles was necessary to predict these features then the two downstroke power muscles are acting as a synergy.
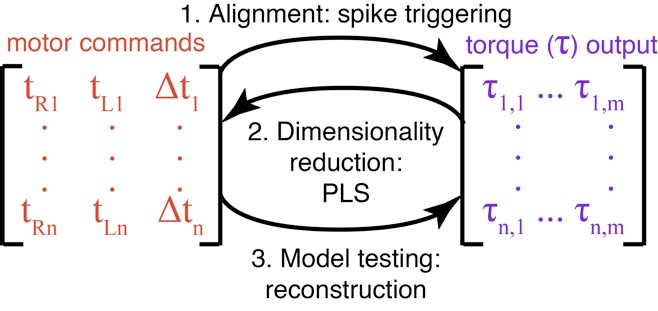
Projection onto Latent Structure (PLS) Feature Analysis: Identifying patterns in torque production that covary with changing motor commands
Unlike a principle components approach which identified upwards of eight different features of torque that could be important, our partial least squares (PLS) feature analysis captures all the variation in torque that is affected by changes in downstroke muscle timing in only two significant features. More generally, the PLS feature analysis provides a method for dimensionality reduction in neural encoding/decoding questions when only some of the variation in the output signal is driven by the changes in the input and when both input and output are described by many variables. Moreover, while a classical analysis of the muscles’ timings suggested that the two downstroke muscles behave as a synergy, in which only their timing difference is important, our PLS approach rejected this hypothesis. When we instead used the task-relevant motor output features from the PLS analysis, we discovered a significant effect of each muscle on torque production. Therefore, while classical biomechanical analyses using summary statistics predicted that the downstroke flight muscles act as a synergy during yaw turning, our new PLS feature analysis reveals a unique, non-synergist contribution from each muscle.