Animal locomotion poses many challenges to an animal’s sensorimotor processing, especially for goal-directed tasks in dynamic and uncertain environments. To understand these challenges, some neural network models can predict firing rates or other continuous representations of neural parameters or can classify sensory stimuli and motor responses. These models are integrated with traditional motor control models that typically operate in the domain of continuous signals such as muscle forces, muscle activation, and limb and body kinematics. However, they do not capture a key aspect of neural signals essential for movement: temporal precision of spikes and coordination of neural activity. There is growing and ubiquitous evidence that neurons across the sensorimotor cascade encode information in the precise timings of spikes – not just for sensory information encoding and processing, but for the generation of motor behavior as well. Given time-dependencies, precision, completeness, and coordination in the
hawkmoth’s flight motor program, here we explore modeling approaches in which sensory encoding models are integrated with either artificial or spiking neural networks to predict temporally precise comprehensive motor program of the hawkmoth. Beyond predicting a coordinated set of motor spikes for an agile sensorimotor task, such a model can potentially be a critical component of a closed-loop neuromechanical framework that captures precise timings of neural signals not just to encode information and accomplish coordination but also to make causal and context-dependent predictions about locomotion.
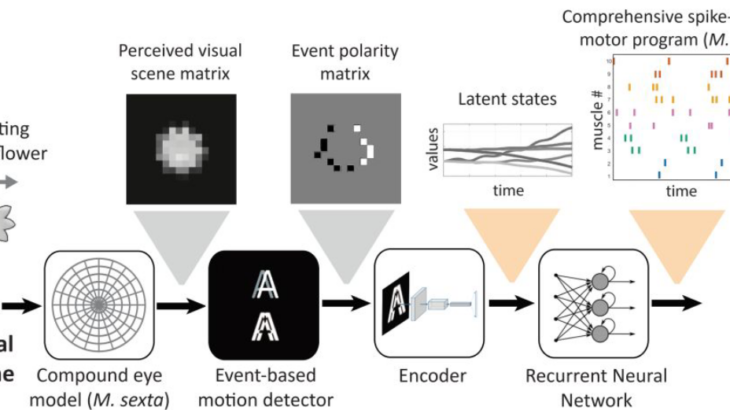